The Benefits of Machine Learning in Manufacturing
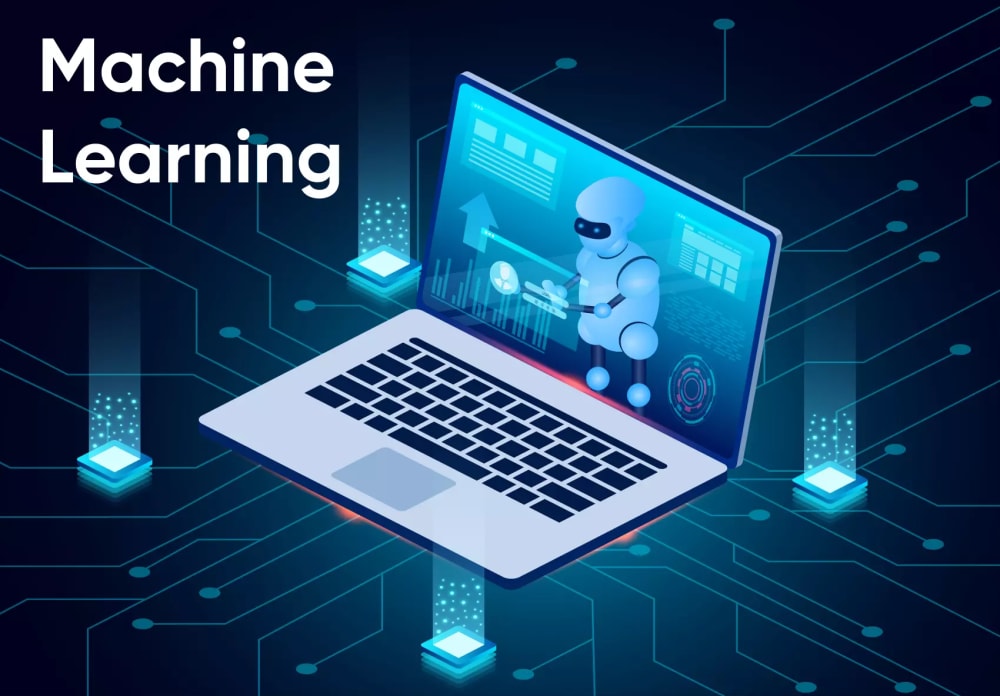
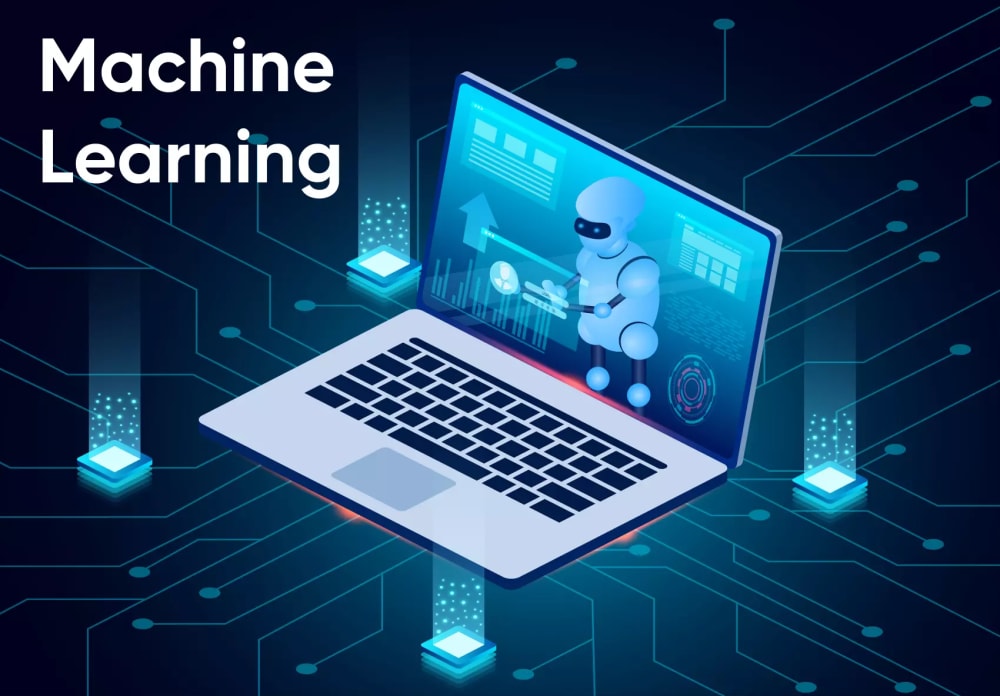
How to Apply ML Algorithms For Quality Control
The modern world requires business leaders and companies to utilise the vast world of digital technologies if they want to stay competitive. Large manufacturers must ensure that their product quality is high and maintains certain attributes.
Today, artificial intelligence and machine learning are helping to develop what is known as smart factories, in particular, these are widely used in the manufacturing industry to improve and speed up the production process. There are many possible machine learning solutions, and it is vital to have confidence in the consultancy of your software development partner.
Go Wombat is currently working on a fascinating, large, and complex project that includes the use of Machine Learning for quality control in various production plants, as part of their overall digital transformation. For this reason, we feel it is good to explain how Artificial Intelligence and machine learning can be applied during the process, the advantages of machine learning in manufacturing, and the steps to use this technology to the full extent. This article will detail the steps taken to develop the project and highlight some of the benefits of machine learning in manufacturing.
How Machine Learning Enhances Manufacturing Processes
According to Fortune Business Insights, the global 'AI in the manufacturing industry's market size is projected to reach USD 9.89 billion by 2027. In contrast, it stood at USD 1.82 billion in 2019. So there are substantial benefits of machine learning in the manufacturing process.
Predictive maintenance
Using predictive maintenance through predictive analytics, machine learning algorithms enable enhanced maintenance in the manufacturing industry by predicting possible failures. Thus, specialists can identify the right time for maintenance and avoid equipment failure or unnecessary downtime since it leads to financial losses and affects the output. This form of monitoring of possible routine tasks and machine maintenance help detect excessive wear and tear in the early stages. Due to machine learning, it is possible to schedule maintenance effectively, bringing the phrase 'Prevention is better than cure' into focus.
Efficient Inventory management
Machine learning makes it possible to automate various logistics, inventory management, and supply chain management tasks, increase efficiency and reduce expenses. The reduction of manual tasks leads to higher employee productivity and improves resource management overall within the manufacturing industry.
Quality control
One of the most valuable and vital fields where artificial intelligence and machine learning can be applied is product quality. This form of control not only refers to the manufactured product but to the equipment failures too. Within this analysis could also be the efficiency of the production process or the energy costs of a specific machine or process. Machine learning algorithms can use specific patterns to analyse manufactured products and detect possible defects. A machine learning network is permanently learning, so it can improve its defect detection process and increase detection rates exponentially.
Development process
The product development process cannot neglect the use of machine learning since it contributes to higher product quality. For example, artificial intelligence and machine learning help collect and analyse vast product data to identify the demand and open new business horizons. As a result of this big data, companies may manufacture products that meet consumer demand with greater effect. So the risk is lowered, and the product is built considering many essential factors. However, even with the best algorithm, predictive quality analytics will only be as effective as the historical data and quality of real-time data that is used in training it. In order to claim success, manufacturers must have a well-designed data collection strategy that captures all relevant information about their process.
Robotics and Machine Learning Algorithms
The integration of machine learning algorithms into robotics used in manufacturing can help reduce the workload and increase the quality inspection level. Furthermore, it is possible to use advanced machine learning techniques in robotics, and involved specialists can build more complex strategies for manufacturing process enhancement. The improved ability to process data analytics is incomparable to human abilities. This side of the operation could include big data, sensor data, or just raw data.
Cybersecurity and Machine learning
The last but not least advantage is the ability to use machine learning in manufacturing to improve digital security, since manufacturers use multiple platforms and tools for data access and processing. Machine learning algorithms can increase the security level and immediately notify specialists in case of any hacking attempts. Some manufacturing systems also include ERP (Enterprise Resource Planning), and SCADA (Supervisory Control And Data Acquisition). These all need protection from outside as well as within.
Need ML-based software? Contact us!
Steps To Get The Most Out Of Machine Learning In Manufacturing
The following steps are necessary to determine when machine learning will fit your manufacturing process adequately and how to use it to the best advantage.
Arrange Proper Data Management
All data analytics to be processed and used by machine learning must be gathered and stored in a single location that will be accessible to machine learning tools. Also, it is essential to relevant data handling platforms which help extract and process big data. In some incidents, big data relating to a project can be stored on cloud servers. At Go Wombat, we are always ready to help you pick appropriate tools.
Identify Your Goals
The next step is to identify the areas of manufacturing where ML could be best used more correctly and where it can quickly deliver value. Here, you need to define your goals one by one and implement selected machine learning models by prioritising tasks by their importance. This is also seen as process optimisation.
Strive For The Maximum Use Of Machine Learning
Even though ML may be required for specific tasks only, you will see the true impact of machine learning on manufacturing processes only when you apply this technology throughout your organisation. So yes, in some areas, ML use will still be beneficial, but we highly recommend applying machine learning to the entire business process in the future.
Enhance Your Staff
Definitely, it is impossible to work with ML-based tools without suitable personnel training and skills. One of your most valuable assets is your workforce, and they need to realise the benefits of machine learning in manufacturing can also benefit them. Therefore, your team should include Data Scientists, analysts, and relevant IT specialists. Staff knowledge and skills are part of the quality improvement felt because of machine learning goals. We consider staff as an integral aspect of the manufacturing business, all-be-it from the more traditional methods, and they are considered and included in all planning.
However, when machine learning is integrated by a software development company like Go Wombat, you can entrust the maintenance process to us. In this case, we will be in charge of product functionality and performance. In addition, we consider all aspects, including the security of any information and data collected and stored either on-site or, more commonly, cloud-based storage.
At the same time, you need to create a data-driven culture in your organisation to convince and explain to personnel the value of ML potential and its significant impact on the entire business. It can be difficult but beneficial to introduce staff to the prospect of artificial intelligence and machine learning solutions.
Contributing To The Quality Assurance In Manufacturing
The Project
Go Wombat received an exciting offer to take part in improving a project built for the manufacturing sector. The project was in the PoC stage (Proof of Concept), and our task was to modify this product.
Background
Some factories create electronic boards with specific components, and components may be created by other factories. However, some dishonest vendors in the market can sell used components under the guise of new ones. Therefore, some factories can purchase damaged or used components. This is where artificial intelligence (supervised machine learning) can come into play by identifying such issues.
Goal
Go Wombat’s development team, and the client’s developers have created trained neural networks using machine learning neurons in a bid to enable process optimisation.
They train these artificial neural networks to detect many aspects, including recognising components and their manufacturers and detecting any defects such as soldering issues. Due to the availability of this information, factories that manufacture electronic boards can understand a specific set of components is either counterfeit or low-quality and the system should discard it.
How It Works In Detail
There is a package of components that should be assembled onto boards. The package falls into two types — ‘reel’ (a blister package containing components within the reel) and ‘tray’ (large electronic boards are stored on these trays). Reels are the most widely used.
There is a manufacturing line consisting of several assembly machines. Reels are inserted into slots in each assembly machine where a mechanical arm pulls out individual components. The machine photographs components before soldering them onto electronic boards, taking only a few seconds. Our software receives photos from the entire line, processes them, and sends a report with statistics and results.
This enables factories to realise in advance whether they can use these components for manufacturing. So the project does two tasks — scanning all components installed on electronic boards, and analysing reels before components go to production.
How much does it cost to create AI-based software? Contact Go Wombat to get a detailed estimate.
Interface And Architecture
The project is a web-based interface. We create a customer’s profile (for a specific factory) in the system, grant rights, and they can monitor their production part. The system has multiple filters, allowing customers to see the information by each reel, ensuring quality control.
For example, they can check all reels that contain damaged or used elements. Or they can see all boards with destructive components. According to Forbes, machine learning-based automated quality testing can increase detection rates by up to 90%. Machine learning-based solutions can automate various logistics-related tasks and manufacturing operations, increasing efficiencies and reducing costs.
We used AWS serverless technology partially, such as AWS Fargate (a serverless core that requires payment upon request, so we pay for this only when service is used). It helps us launch multiple Docker containers on-premise, making the architecture more scalable and cost-efficient. Not all factories work on weekends, and the software doesn't process the same amount of data as on weekdays. So on weekends, all these capacities are reduced, and there is no need to pay for unused resources.
Under normal conditions, this high-load system can process a few million components per day.
All factories have different internal structures, so it is necessary to customise the configuration for each customer. Each factory requires a custom approach (a particular section might require the installation of an additional server, while others want us to help them deal with network issues), so there are many nuances. We need to build the data-gathering process from the customer’s factory, and then we configure the access to the software.
It is not an easy task to create a single system for everyone. Factories can be similar, but they all have different internal organisations and requirements. Even one giant company with many factories worldwide will require a custom approach for each factory since standards vary depending on the region.
Challenges
Our development team spent a lot of time identifying how the software should work and, in fact, how the entire system works. Developers had to delve into each process to create the app and understand the manufacturing technology. Furthermore, large factories have a lot of documentation, and our specialists have to get into these processes, making us thankful for efficient inventory management.
Also, considering that the project is constantly changing and improving, the effect of new staff from both sides of the project creates additional strains through onboarding.
Our current task is to extend the standards to ensure that more factories can use the software.
Tech Stack
Of course, Python was the core tool for back-end development. The back-end section was created initially using C# and F# before we started working on it. Also, we used a versatile and convenient Fast API framework to create APIs quickly.
Their developers initially created the machine learning network, but we have integrated it into the system and used TensorFlow, Keras (both of them are libraries), and PyTorch (framework). At the moment, we use TensorFlow only. These tools are all Python-related since Python is considered the most suitable language for AI and ML.
As mentioned previously, we used AWS and AWS Fargate for cloud infrastructure. Serverless technologies were used partially, but we plan to use them more in the future. We migrate our API using serverless technologies, and this API is used for data acquisition on the front-end. We also used AWS SQS as an intermediary between microservices.
Also, we used CI/CD as a DevOps technology. It stands for Continuous Integration and Continuous Development. This enables uninterrupted code integration and automated code analysis negating the need to deploy the code manually.
Result
We have been working on this project for over one year. Go Wombat entirely developed the cloud aspect. Previously, it was a Proof of Concept, as we mentioned initially. We have connected all software elements and built a full-fledged product.
This project is set to be of huge benefit to the manufacturing industry and supply chain management with the detailed design of the process. Any production line quality, accuracy, and efficiency will see improvement.
Unlock Success with Premium Software Development
Contact us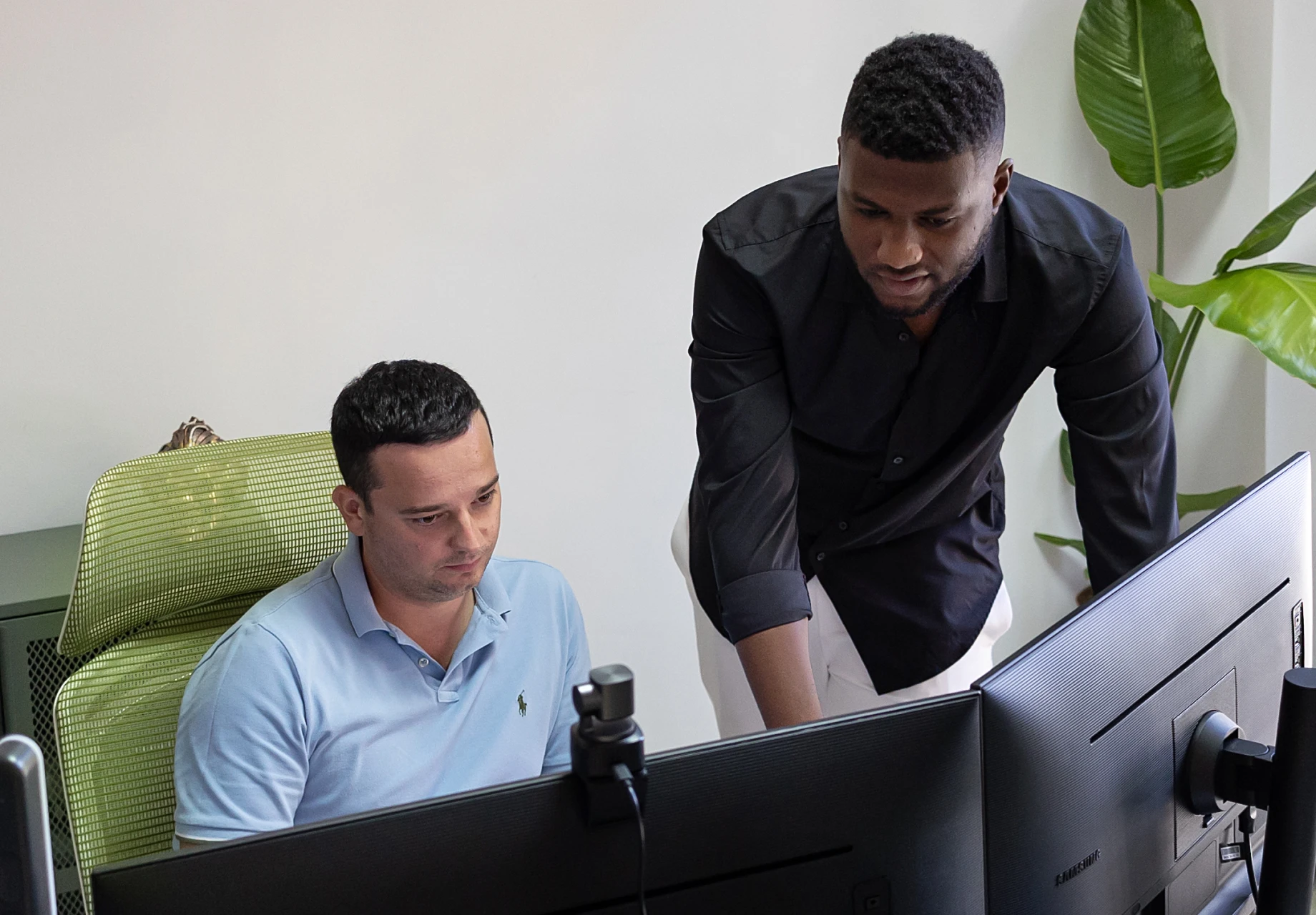
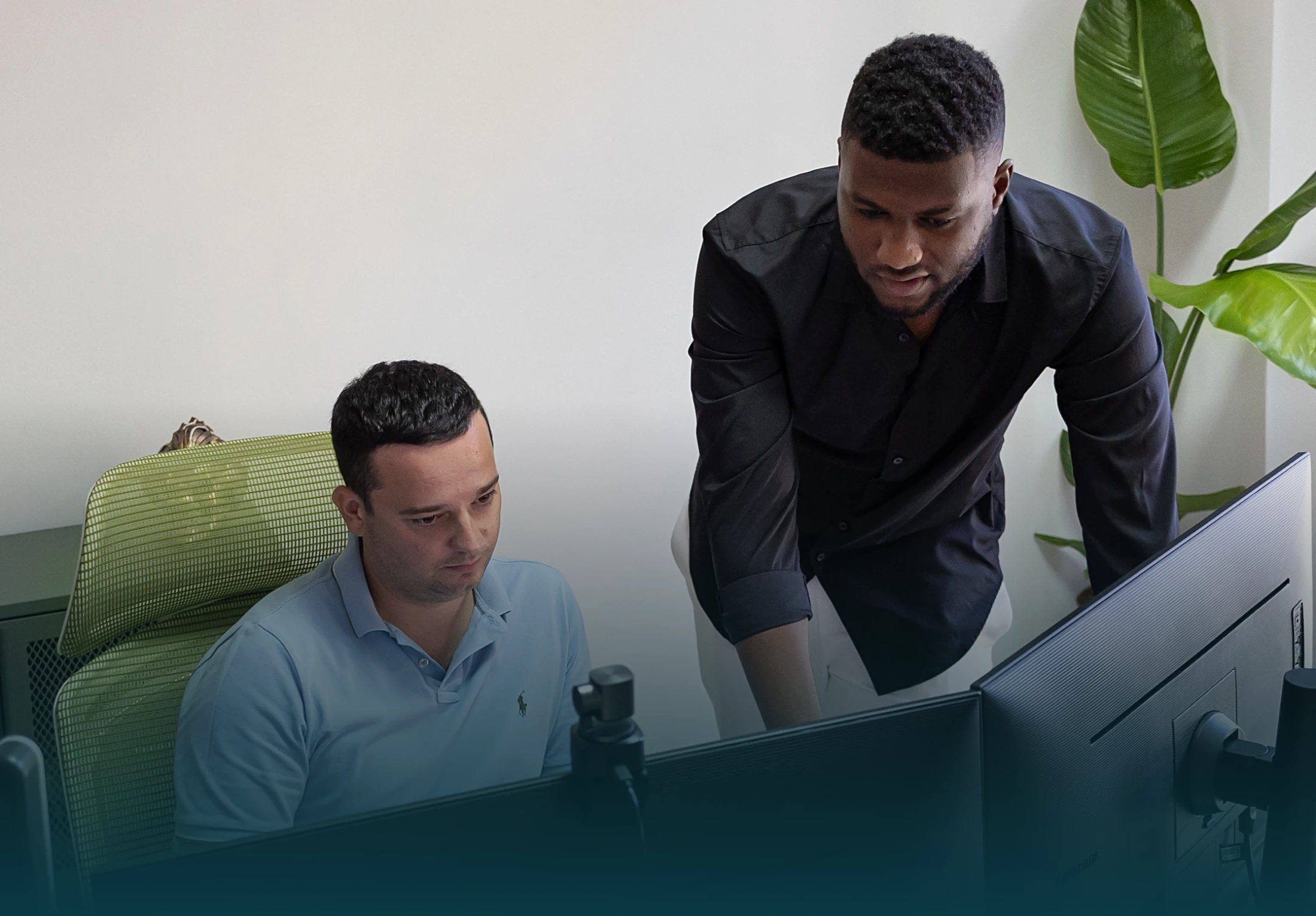
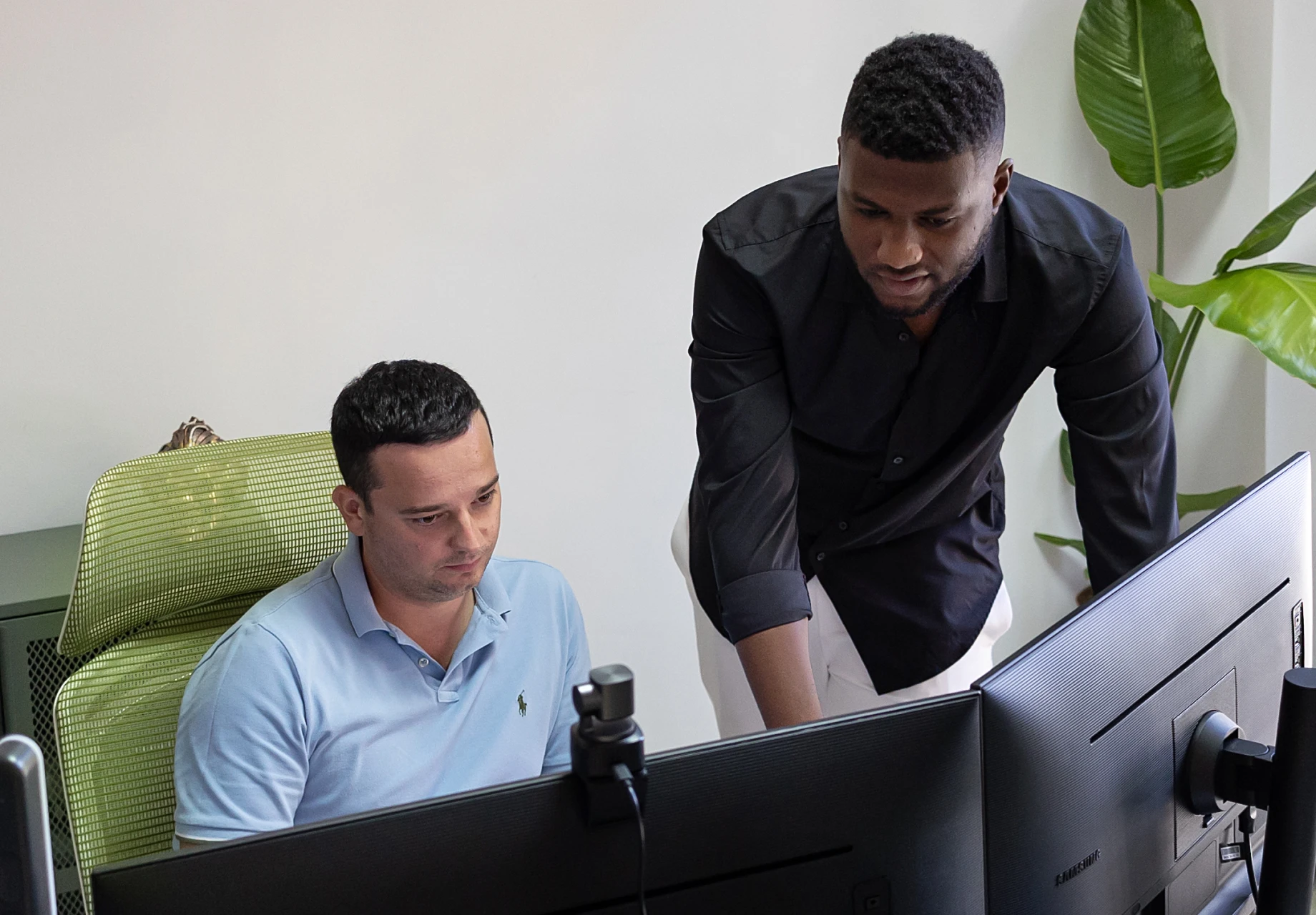
Conclusion
The use of machine learning modifies any sector, not only the manufacturing industry. At Go Wombat, our developers continually monitor up-to-date trends in the software development world to make sure that your new software will be competitive and contemporary.
The “Smart Manufacturing” revolution is already enabling manufacturers to achieve this goal, and one of the core technologies at the center of this new wave of innovation is Industrial Artificial Intelligence and Machine Learning.
The idea that smart factories were once just car manufacturers with industrial robots has long been forgotten, and the manufacturing sector, as a whole, is now embracing new business opportunities.
The project we are working on is very complex, and it takes a lot of effort from our developers. However, it helps them increase their skill level continuously and achieve new heights. Furthermore, it is impossible to learn something new without facing challenges.
We welcome the opportunity to help you build the proper tool for your business. By sharing your vision with us, you are stepping into a world of expansion, increased productivity, and the global smart manufacturing market.
Go Wombat is a dedicated and professional software development company. Contact us to discuss your smart manufacturing needs for a section or your entire production process.
How can we help you ?
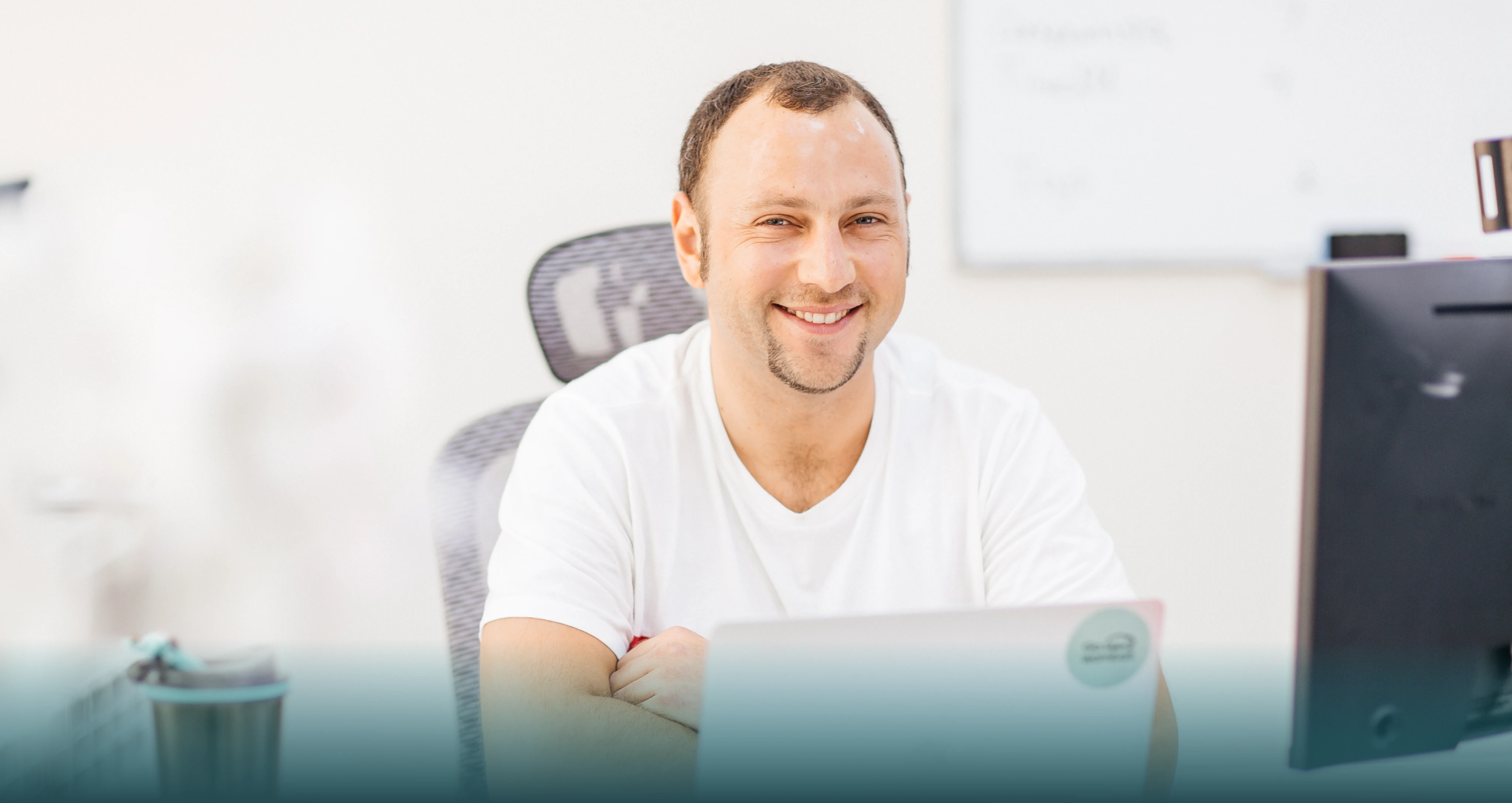
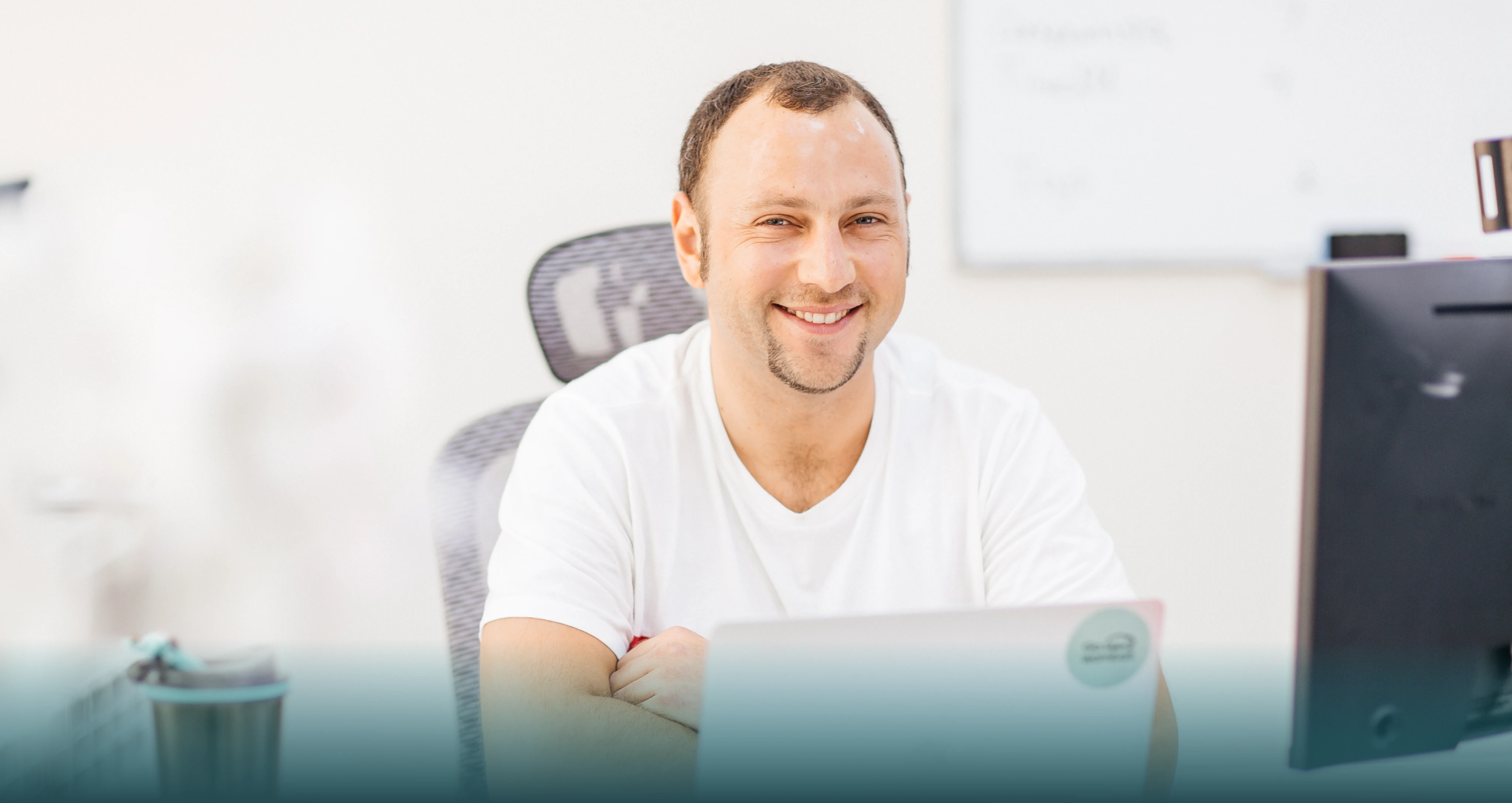
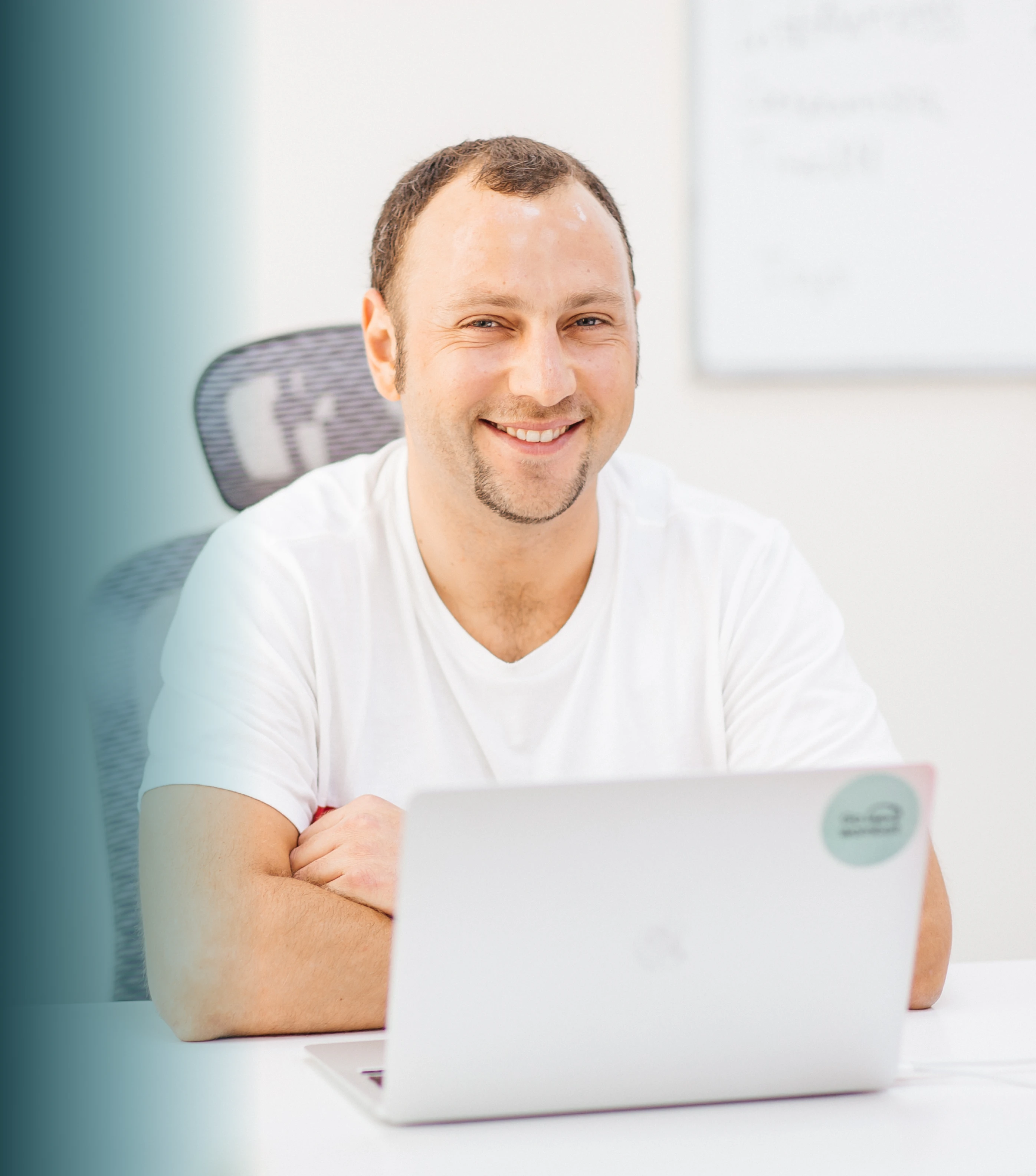